- Self-administered versus Clinician-performed BinaxNOW COVID Rapid Test: A Comparison of Accuracy
These are the results of a single-center study at a free community testing site in Baltimore City to assess the accuracy of self-performed rapid antigen tests (RATs) for COVID-19. Self-administered BinaxNOW RATs were compared with clinician-performed RATs and against a reference lab molecular testing as the gold standard. Of the 953 participants, 14.9% were positive for SARS- CoV-2 as determined by RT-PCR. The sensitivity and specificity were similar for both self- and clinician-performed RATs (sensitivity: 83.9% vs 88.2%, P = 0.40; specificity: 99.8% vs 99.6%, P = 0.6). Subgroup comparisons based on age and race yielded similar results. An interesting issue was that 5.2% of positive results were potentially missed due to participant misinterpretation of the self-test card. The test did very well in symptomatic individuals with self- and clinician-performed RATs (sensitivity: 88% vs 90%)
- The Impact of COVID-19 Vaccination Prior to SARS-CoV-2 Infection on Prevalence of Long COVID among a Population-based Probability Sample of Michiganders, 2020-2022
Authors used data from the Michigan COVID-19 Recovery Surveillance Study, a population-based probability sample of adults with COVID-19 (n=4,695). They considered 90-day Long COVID (illness duration ≥90 days). They compared vaccinated (completed an initial series ≥14 days before COVID-19 onset) to unvaccinated individuals (received 0 doses before COVID-19 onset), accounting for differences in age, sex, race and ethnicity, education, employment, health insurance, and rurality/urbanicity. The full unvaccinated comparison group was further divided into historic and concurrent comparison groups based on timing of COVID-19 onset relative to vaccine availability. Here we get prevalence ratios (PRs). Compared to the full unvaccinated comparison group, the adjusted prevalence of 90-day Long COVID was lower among vaccinated individuals PR90-day=0.42(95%CI:0.34,0.53)]. So that gives us a 47-66% lower prevalence of Long COVID at 90 days in the vaccinated. Estimates were consistent across comparison groups (full, historic, and concurrent).
- Estimates of Incidence and Predictors of Fatiguing Illness after SARS-CoV-2 Infection
This is a retrospective cohort analysis. Investigators analyzed electronic health records data of 4,589 patients with confirmed COVID-19 during February 2020–February 2021 who were followed for a median of 11.4 months and compared this data to data from 9,022 propensity score–matched non–COVID–19 controls. This data was collected from the University of Washington (UW) that included 3 hospitals (Harborview Medical Center, UW Medical Center Northwest, and UW Medical Center Montlake) and >300 primary care and specialty clinics providing healthcare services across the state of Washington, USA. Fatigue was defined by any of the following ICD-10-CM or International Classification of Diseases, 9th Revision, Clinical Modification (ICD-9-CM), diagnostic codes recorded in EHR during the postacute period: G93.3, postviral fatigue syndrome; R53.82, chronic fatigue, unspecified; R53.83, other fatigue; 780.71, chronic fatigue syndrome/postviral fatigue syndrome; or 780.79, malaise and fatigue. They defined incident fatigue as a patient who had >1 diagnostic code for fatigue during the postacute period. This is dependent on the treating provider not just recognizing the fatigue but adding this to the coding for that visit. Among COVID-19 patients (15% hospitalized for acute COVID-19), the incidence rate of fatigue was 10.2/100 person-years and the rate of chronic fatigue was 1.8/100 person-years. Compared with non–COVID-19 controls, the hazard ratios were 1.68 (95% CI 1.48–1.92) for fatigue and 4.32 (95% CI 2.90–6.43) for chronic fatigue. The observed association between COVID-19 and the significant increase in the incidence of fatigue and chronic fatigue reinforces the need for public health actions to prevent SARS-CoV-2 infections. - Long-term risks of Respiratory Diseases in Patients Infected with SARS-CoV-2: A Longitudinal, Population-based Cohort Study
This is a longitudinal, population-based cohort study, where they built three distinct cohorts age 37–73 years using the UK Biobank database; a COVID-19 group diagnosed in medical records between January 30th, 2020 and October 30th, 2022, and two control groups, a contemporary control group and a historical control group, with cutoff dates of October 30, 2022 and October 30, 2019, respectively. The follow-up period of all three groups was 2.7 years. They included 112,311 individuals in the COVID-19 group with a mean age (±SDs) of 56.2 (8.1) years, 359,671 in the contemporary control group, and 370,979 in the historical control group. They found elevated Hazard ratios for a number of different respiratory disorders in the COVID-19 group. They reported for asthma a HR of 1.49; bronchiectasis (1.30); COPD (1.59); ILD (1.81); pulmonary vascular disease (PVD) (1.59); and lung cancer (1.39). Notably, the authors suggest, “our study found a significant association between COVID-19 and lung cancer, but we fully recognize that this may be due to large-scale chest CT scans performed on a large proportion of suspected or confirmed patients with COVID-19, leading to more early tumor cases being detected. Therefore, clinically, more caution is required when interpreting the association between them.” Some discussion about the mechanism for the other respiratory issues, and then they conclude, “Our research suggests that patients with COVID-19 may have an increased risk of developing respiratory diseases, and the risk increases with the severity of infection and reinfection.”
Situation Dashboards
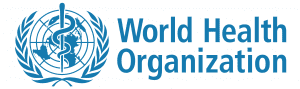
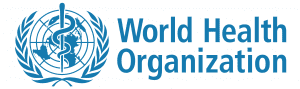
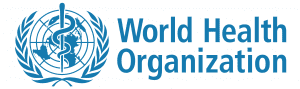
World Health Organization (WHO)
Novel Coronavirus (COVID-19) Situation from World Health Organization (WHO)


Johns Hopkins University (JHU)
Coronavirus COVID-19 Global Cases by the Center for Systems Science and Engineering (CSSE) at JHU
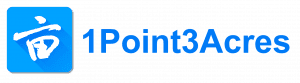
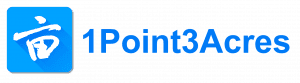
COVID-19 in US and Canada
1Point3Acres Real-Time Coronavirus (COVID-19) Updates in US and Canada with Credible Sources
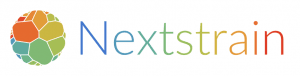
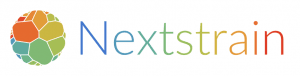
Genomic Epidemiology COVID-19
Genomic Epidemiology of (COVID-19) Maintained by the Nextstrain team, enabled by data from GISAID.